Geospatial analytics lies at the core of spatial computing, enabling the gathering, processing, and analysis of data with a geographic or locational component. This cluster explores how geospatial analytics enhances spatial computing applications, its role in various industries, and how it integrates with key technologies like digital twins, AR & VR, and future tech.
Listen to our podcast: Geospatial Analytics & Location-Based Services: The Backbone of Spatial Computing
What Is Geospatial Analytics?
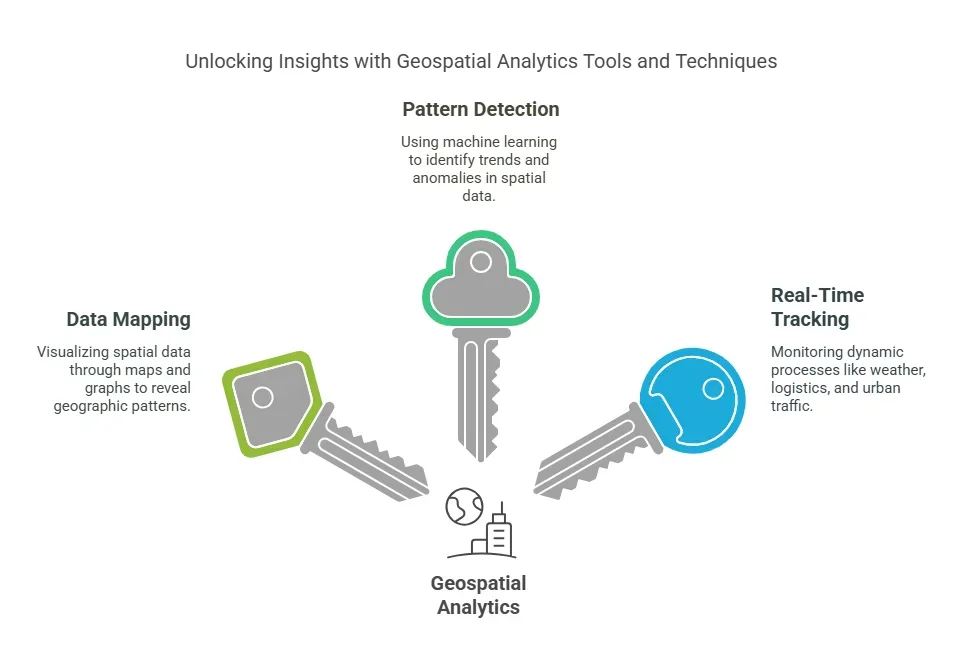
Geospatial analytics uses tools and techniques to analyze data tied to a specific location. Through the use of geographic information systems (GIS), GPS, satellite imagery, and AI-powered mapping software, geospatial analytics provides actionable insights into real-world phenomena.
Key Features of Geospatial Analytics
- Data Mapping: Visualize spatial data through maps and graphs.
- Pattern Detection: Identify trends and anomalies using machine learning.
- Real-Time Tracking: Monitor dynamic processes like weather patterns, logistics, and urban traffic.
For an overarching perspective, check out our Spatial Computing Pillar Blog to see how geospatial analytics fits into the bigger picture.
Applications of Geospatial Analytics
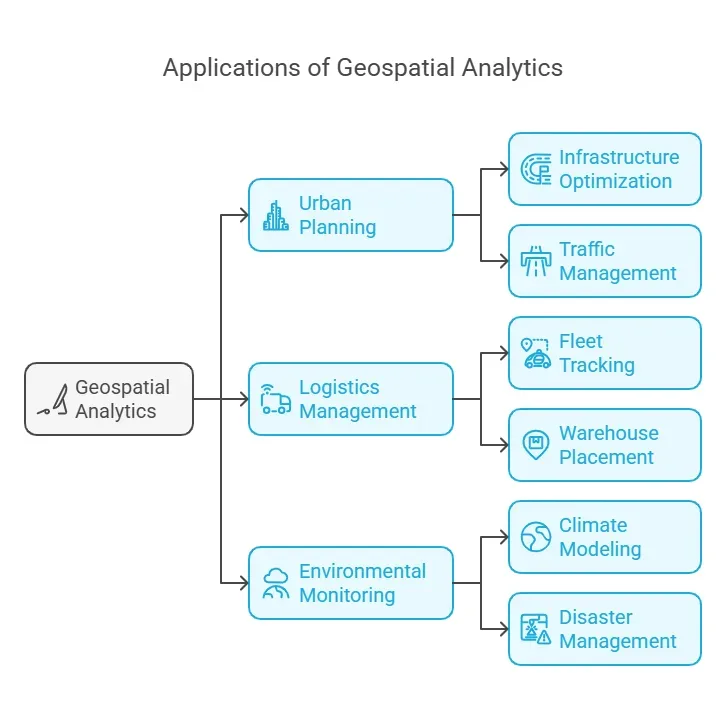
Geospatial analytics powers innovation across a wide range of industries by providing critical insights into spatial data.
Urban Planning and Smart Cities
- Infrastructure Optimization: Streamline urban utilities like power grids, water supplies, and public transport.
- Traffic Management: Analyze traffic flows to design more efficient road networks.
Learn how geospatial analytics supports Digital Twins & Real-World Simulations in creating smarter cities through real-time urban planning insights.
Logistics and Supply Chain Management
- Fleet Tracking: Real-time GPS monitoring ensures efficient routing and reduced delivery times.
- Warehouse Placement: Determine optimal locations for storage facilities based on demand patterns.
See how geospatial analytics integrates with AR & VR Innovations to create immersive dashboards for real-time logistics visualization.
Environmental Monitoring
- Climate Modeling: Map climate changes and predict their impact on ecosystems.
- Disaster Management: Aid in disaster preparedness and recovery by identifying vulnerable areas.
For insights on future capabilities, explore Future Trends & Emerging Tech to see how advancements like AI and edge computing enhance geospatial data processing.
Technical Framework of Geospatial Analytics
Geospatial analytics relies on a robust infrastructure combining data collection, processing, and visualization technologies.
Data Sources
- Satellite Imagery: High-resolution visuals for mapping large areas.
- IoT Sensors: Ground-level data for localized insights.
- Drones: Aerial data collection for precise and real-time updates.
Integration with Spatial Computing
Geospatial analytics complements technologies like digital twins and AR & VR by providing context-aware data layers for more informed decision-making.
Challenges and Ethical Considerations
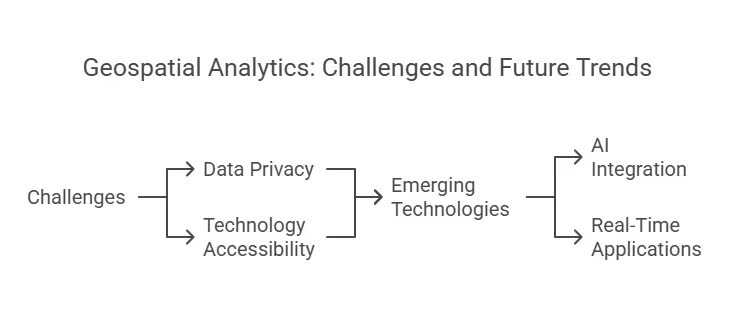
As with any advanced technology, geospatial analytics has its own set of challenges and ethical dilemmas.
Data Privacy
- Protecting sensitive geographic data, especially when linked to personal identifiers, is crucial.
- Adhering to global data protection regulations ensures ethical use.
For detailed strategies, refer to Security, Privacy, and Ethical Considerations.
Accessibility of Technology
- Advanced geospatial tools may be inaccessible to smaller organizations due to high costs.
- Open-source solutions can democratize access to geospatial analytics.
Future of Geospatial Analytics in Spatial Computing
The future of geospatial analytics is being shaped by emerging technologies like AI, 5G, and edge computing, which are making spatial data processing faster and more accurate.
Emerging Trends
- AI Integration: Automate pattern recognition and predictive analytics for more efficient data processing.
- Real-Time Applications: Low-latency processing through 5G and edge computing enables immediate insights.
Explore the cutting-edge possibilities in Future Trends & Emerging Tech.
Conclusion
Geospatial analytics serves as the backbone of spatial computing, enabling location-based insights that drive smarter decision-making, optimize processes, and enhance user experiences across industries. By integrating with technologies like digital twins and AR & VR, geospatial analytics delivers a context-aware approach to spatial data. With advancements in AI and edge computing, its potential is only expanding, making it an indispensable tool for businesses, governments, and organizations aiming to stay ahead in an increasingly data-driven world.
FAQ
Q1: How does geospatial analytics enhance spatial computing?
Geospatial analytics provides the location-based insights that power spatial computing, enabling smarter decisions and enhanced user experiences.
Q2: What industries benefit most from geospatial analytics?
Industries like urban planning, logistics, environmental monitoring, and disaster management leverage geospatial analytics to optimize operations and minimize risks.
Q3: How does AI improve geospatial analytics?
AI enhances geospatial analytics by automating data analysis, recognizing patterns, and enabling real-time predictive insights.
Q4: What are the ethical challenges of geospatial analytics?
Ethical challenges include protecting sensitive data, avoiding misuse of location-based information, and ensuring equitable access to the technology.
Q5: How does geospatial analytics integrate with digital twins?
Geospatial analytics provides the data layers that enable digital twins to simulate and analyze real-world environments in real time.
6 thoughts on “Spatial Computing & Generative AI: Transforming the Future of Digital Experiences”