Data integration is vital for using digital twin technology. Digital twin helps to enhance the organization’s performance, monitor the reliability, maintain assets, and optimize the operation. However, as soon as it comes to manage and integrate multiple information flows from different systems, they are struggling heavily. In this article, we will discuss the major data integration issues in digital twin applications. We will also discuss the different ways that will enable us to overcome the above challenges.
Listen to Our Podcast: Overcoming Data Integration Challenges in Digital Twin Implementation
Understanding Data Integration in Digital Twin Technology
Data integration refers to the aggregation of data from multiple sources into the one that provides the whole picture. In the case of digital twins, the process is critical. It makes sure that information collected from the sensors, IoT devices, and other working systems is synchronized to the physical entity. Such accuracy is especially important as it makes it possible to carry out continuous analysis, modelling, and optimization of the process.
However, there is always some issue when it comes to data integration in the case of a digital twin. Original problems may arise from the nature of data, difficulty in analyzing large data, and variations in data structure. Moreover, real-time integration between single systems is required. Addressing these issues on the side of organizations will assist in enhancing the advantages of digital twin technology on the side of organizations.
Key Data Integration Challenges in Digital Twin Technology
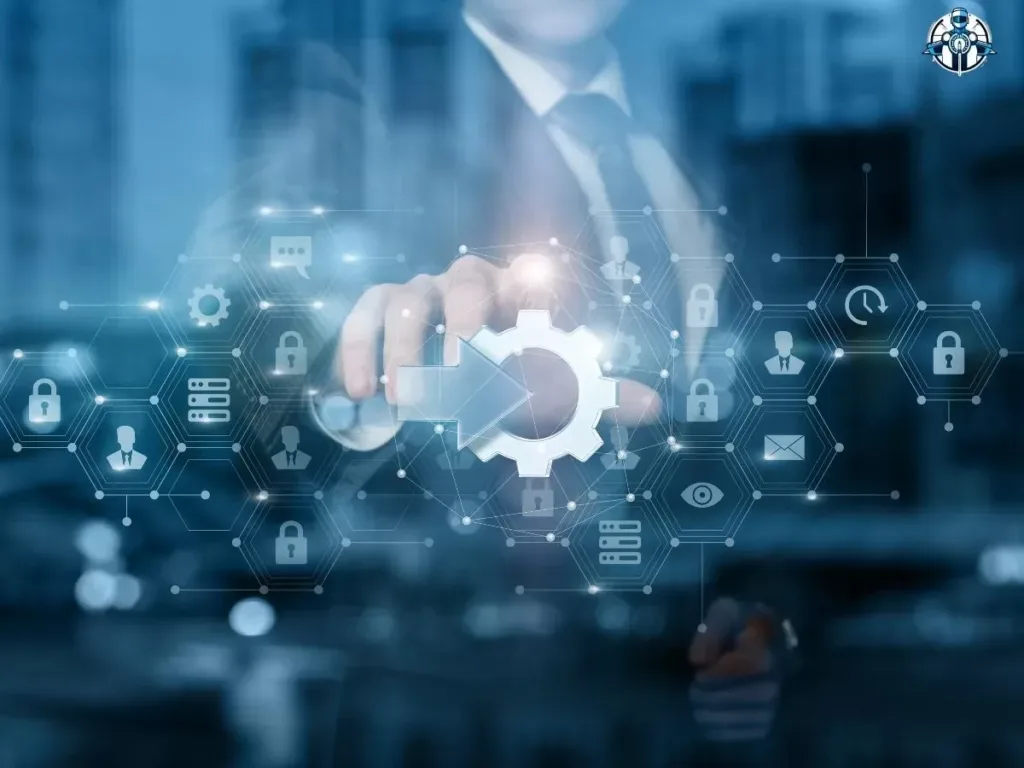
Several challenges need to be addressed to ensure effective data integration in digital twin implementations:
· Data Variety
Digital twins extract information from several databases. These include; Sensors, Databases, and Enterprise Systems. Each source has different formats, protocols, and structures. This variety makes it difficult to combine and process the data.
· Data Quality
To be effective, digital twins depend on having high-quality data. The data must be accurate and reliable as well as valid. If the data is wrong, missing, or outdated one can make wrong decisions in controlling the business. This can lead to wrong simulation models and wrong predictions are made.
· Real-Time Data Processing
Digital twins require the use of real-time data. They use this data to make the right decision at the right time. Call records are sometimes voluminous and managing as well as processing colossal data in real time is not easy. This is especially the case especially when one is dealing with big quantities of data.
· System Interoperability
Organizations often use different software tools in various departments. These systems need to communicate effectively. Ensuring their compatibility is important. If systems cannot work together, data silos can form. This disrupts the integration process.
· Security Concerns
Digital twins use large amounts of data. Some of this data is sensitive or proprietary. It is important to secure data integration. Protecting the digital twin’s integrity matters too. Safeguarding data privacy is also crucial. All these aspects need attention during implementation. Want to learn how predictive maintenance works with digital twins? Discover how it reduces downtime and costs in our guide on Predictive Maintenance with Digital Twins
Strategies to Overcome Data Integration Challenges in Digital Twin Implementation
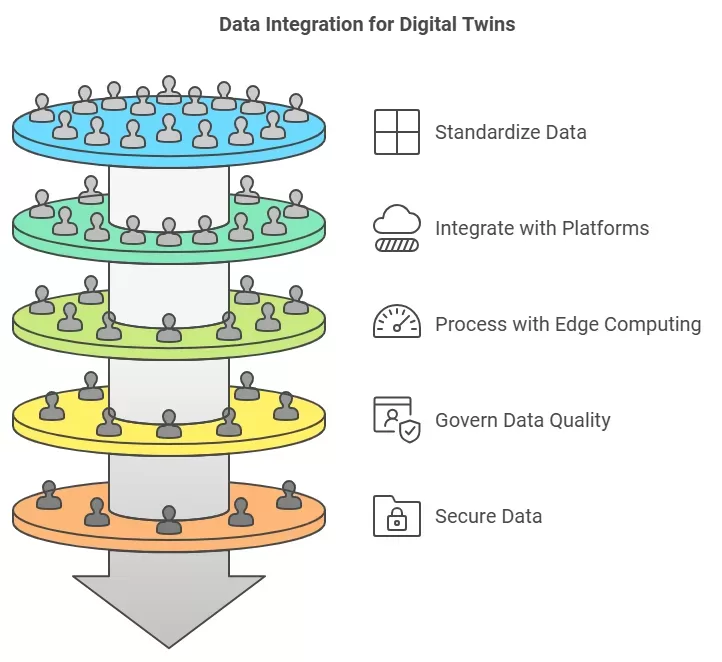
Integrating data into digital twin systems can be tricky. Organizations need effective solutions and clear processes. Here are some key strategies to help overcome these challenges:
1. Implementing Data Standardization Protocols
Standardizing data formats and protocols can solve the issue of data variety. Organizations should implement processes to ensure data structure remains consistent. By using industry-standard data formats like MQTT, OPC UA, and RESTful APIs, they can improve communication between systems.
2. Leveraging Advanced Data Integration Platforms
Organizations can use advanced data integration platforms. These platforms support machine learning and AI. They automate processes for data aggregation and cleansing. They can also manage large amounts of real-time data. This way, the digital twin stays updated with accurate information. Common tools for this include ETL (Extract, Transform, Load) solutions, cloud platforms, and data lakes.
3. Using Edge Computing for Real-Time Data Processing
Edge computing cuts latency. It processes data close to its source. This occurs at the edge of the network, like on IoT devices or sensors. This approach lightens the load on central servers. As a result, it speeds up data flow to the digital twin.
4. Ensuring Data Governance and Quality Control
You should implement data governance frameworks. They ensure the data’s integrity and quality. These frameworks involve validating, cleaning, and verifying the data. Do this before using the data for simulations or decision-making.
5. Addressing Security Concerns with Robust Protocols
Data privacy and security matter greatly. Organizations must use strong cybersecurity protocols. Securely encrypt the data. Use secure APIs and role-based access control. Also, ensure the data transmission is safe. These measures protect data for the digital twin and stop unauthorized access. Explore how digital twins are transforming production processes in our post: Digital Twins in Manufacturing. Uncover the future of efficiency and innovation.
The Role of AI and Machine Learning in Data Integration
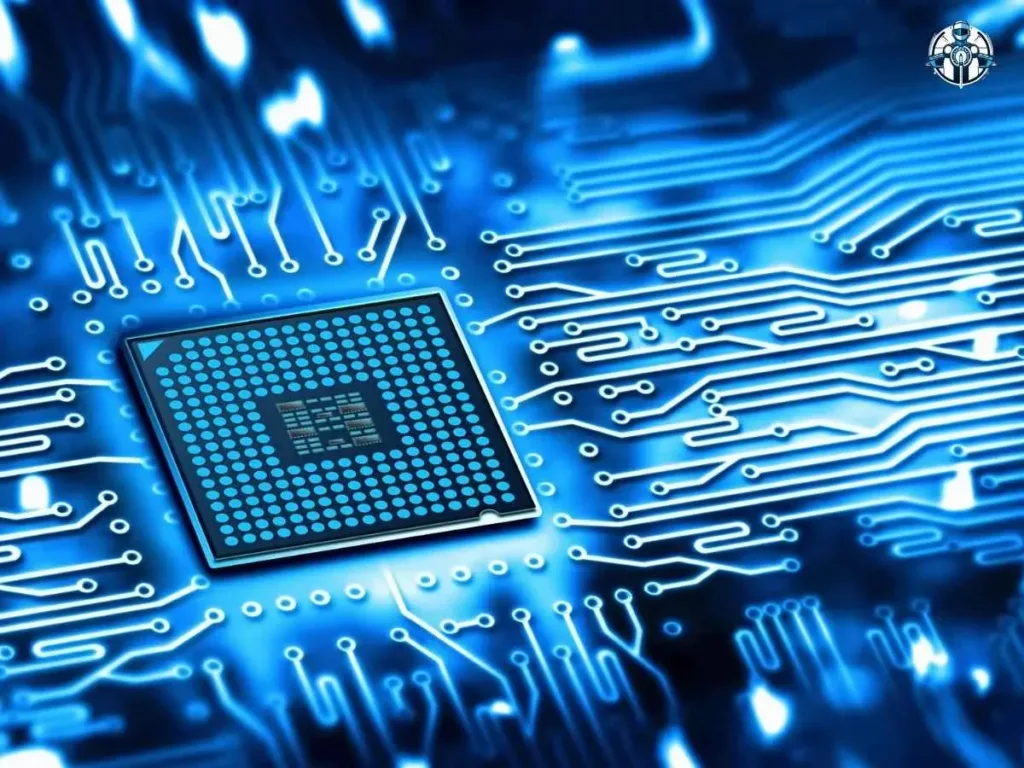
AI and Machine Learning boost data integration for digital twins. They help improve efficiency and accuracy. AI algorithms automatically find patterns, anomalies, and correlations in the data. This ability leads to faster decision-making and better insights.
For example, AI can spot faulty sensor data. It can also identify when the digital twin model does not match real-world data. Additionally, Machine Learning models keep improving the data integration process. They learn from new data streams and refine integration methods continuously.
See how digital twin technology connects the physical and digital worlds. Check out our guide: Digital Twin Technology for deeper insights
Future Trends in Data Integration for Digital Twins
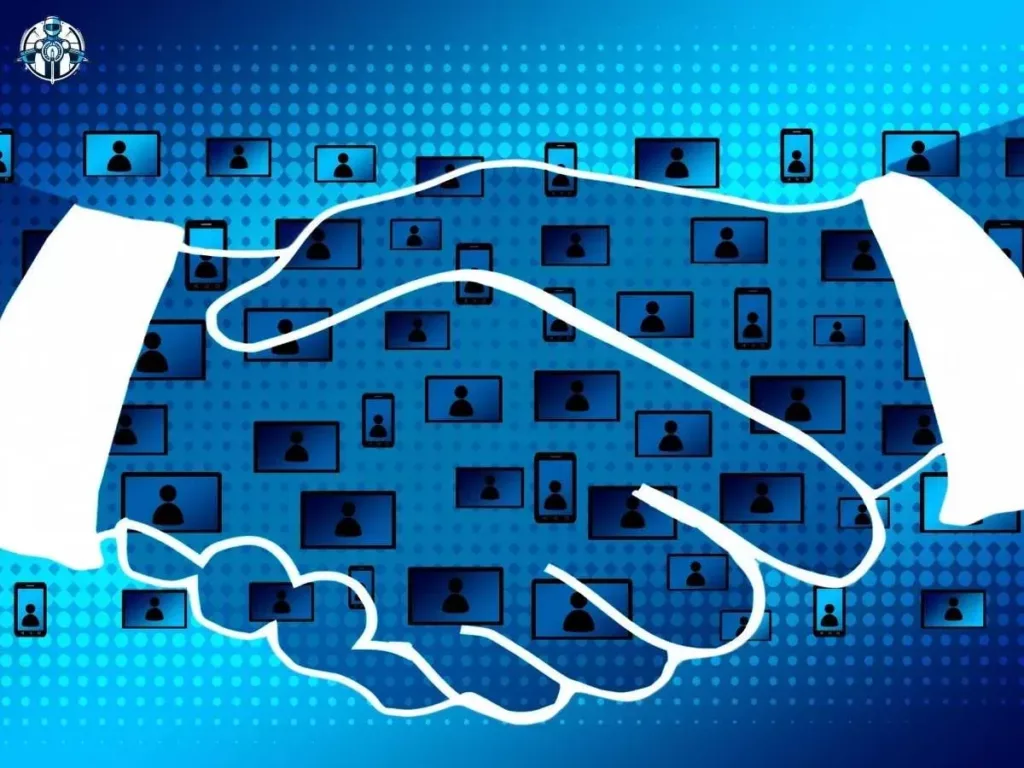
Digital twin technology is evolving rapidly. Several key trends in data integration are emerging.
First, we will see increased use of blockchain for data security. Blockchain can improve data integrity and traceability. It ensures that the data flowing into the digital twin is tamper-proof and securely recorded.
Next, integration with 5G networks is on the rise. The rollout of 5G technology will enable faster and more reliable data transfer. This change will make it easier to integrate and process real-time data from IoT devices and sensors.
Additionally, we will see the rise of advanced data orchestration platforms. As digital twins become more complex, these platforms will manage large-scale data integrations. They will ensure that all systems work well together.
Curious about component and asset twins? Learn their role in digital twin tech in our blog: Understanding Component and Asset Twins in Digital Twin Technology.
Conclusion
Data integration presents a major challenge in implementing digital twin technology. Organizations must address these challenges with the right tools and strategies. This approach will help them ensure accurate, real-time insights that optimize performance and decision-making.
By overcoming hurdles like data variety, quality, processing speed, and system interoperability, companies can fully use the potential of digital twins. This capability can help them reduce costs, increase efficiency, and create innovative new business opportunities. To learn more about how digital twins can transform your operations, explore our other articles, such as the Future of Digital Twin Technology and Digital Twins in Manufacturing.
FAQs
What is data integration in digital twins?
Data integration in digital twins combines data from many sources. It creates a single digital model. This model enables real-time monitoring, analysis, and system optimization.
Why is data quality important for digital twins?
Accurate and consistent data is very important. It ensures the digital twin reflects the physical system. Poor quality data leads to incorrect predictions. This can result in bad decision-making.
How can AI help with data integration for digital twins?
AI can automate the data processing. It can also find anomalies in data. Furthermore, AI learns from new data streams. It improves the integration process and optimizes data aggregation techniques.
What security measures are needed for digital twin data integration?
Robust cybersecurity protocols are essential. Use measures like encryption, secure APIs, and role-based access control. These steps protect sensitive data within digital twin systems.