Think of a possibility where machines are not only able to execute operations they are told to execute. They can think, plan, and make decisions to solve problems. This vision is not just a story—it is the real-world impact of agentic AI. This interesting technology alters the way in which we interact with machines.
According to recent studies, the artificial intelligence market is expected to grow significantly, reaching approximately $1.8 trillion by the year 2030. This reflects an average growth rate of over 36% per year from its current valuation of around $196.6 billion in 2023. This can be attributed to the growth in the application of agentic AI which enhances the intelligence of machines.
In this article, we’ll discuss agentic AI meaning. We will discuss what it is, how it functions, and why it is the future of the entire AI research sphere. Furthermore, we are going to discuss the advantages and disadvantages of using it, how it can be applied, and the problems it may cause. So, let’s get started.
What is Agentic AI?
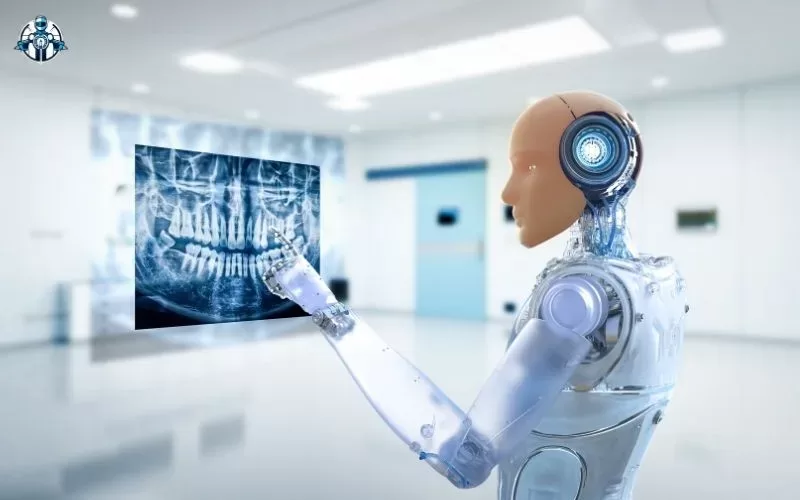
Agentic AI is the next significant development in artificial intelligence. It integrates a number of methods and architectures to synthesize self-directed agents. These agents are able to determine what information is needed, and what goals need to be set and can act accordingly with only minimal human intervention. They are able to reason and find solutions to problems. Like human beings, they change and acquire knowledge on their interactions in different surroundings.
By definition, Agentic AI is the type of AI that can deliberate and execute its decision. It incorporates state-of-the-art language models, machine learning, deep learning, and reinforcement learning. Bots such as ChatGPT assist such systems to comprehend and interpret human language. Machine learning assists them in processing the data and coming up with some patterns. On the other hand, reinforcement learning helps them to learn from the experiences they have gained. Therefore, this helps in enhancing the employees’ capacity for decision-making.
This capability enables autonomous agents to make choices without strict guidelines. This change represents a significant step in technology. Instead of just automating specific tasks, agentic AI aims to make machines helpful partners in solving problems and making decisions. This shift could change many industries and alter how we interact with technology.
Want to know how agentic AI differs from traditional AI? Check out our detailed guide on Agentic AI vs. Traditional AI: Key Differences and Why It Matters to learn more.
How Does Agentic AI Work?
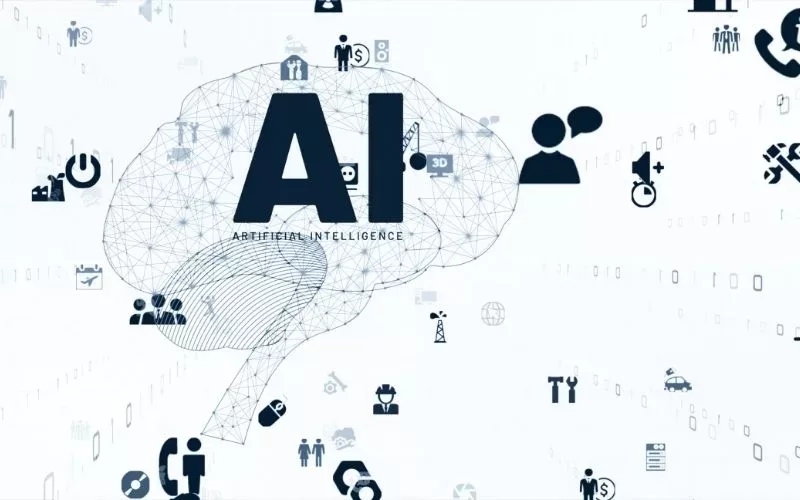
Agentic AI consists of a network of independent software components known as agents. These agents analyze large data sets and learn from user interactions. Over time, their performance improves. Each agent has its specific goals and abilities. They collaborate to tackle complex problems. This system uses advanced technologies, including machine learning and natural language processing. These technologies enable effective learning, communication, and reasoning among the agents.
The structure of the agentic AI is based on a distributed systems platform. This design is scalable, and its performance is quite good. Therefore, the number of agents can work simultaneously across multiple servers. This increases the reliability of the transport system, thus increasing efficiency. The agents are actual, and they exchange information and act synchronously. This results in a seamless interface with the users.
In Agentic AI, self-contained agents are used. These agents are capable of working on tasks on their own as well as handling workflow. They employ artificial intelligence, mathematical models, and pattern recognition. This makes them to be in a position to make decisions with a lot of ease.
What are the Fundamental Principles of Agentic Artificial Intelligence Architecture?
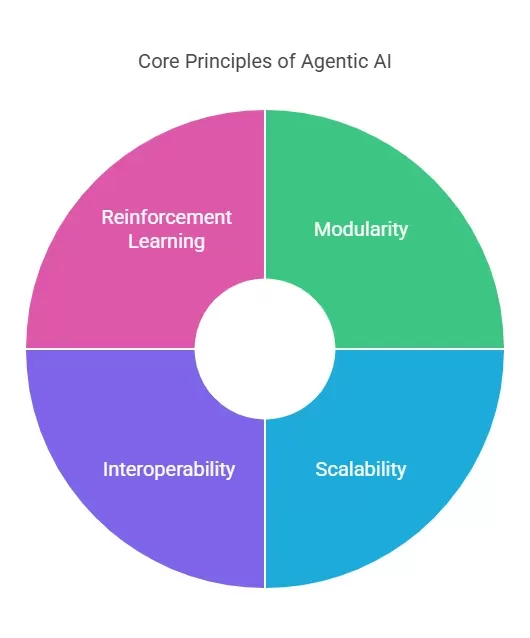
The architecture of the agentic AI is based on the idea of achievement and flexibility in the contemporary advanced technological society. It follows four main principles:
1. Modularity:
This is a concept that divides a large project into smaller categories of subprojects. Every one of them is responsible for some specific tasks like perception or action. This model makes it easier to build, modify or even upgrade a system since there are only a few components. It also assists in enhancing flexibility and enables everyone to easily embrace new technologies.
2. Scalability:
Scalability refers to the ability of an AI system to increase the amount of resources it has. They can take more data and can work with an enhanced level of complexity. Cloud computing and distributed computing technologies can be applied to extend organizations’ architectures. This is because this approach enables them to meet growing needs without compromising the levels of performance. However, they continue to be flexible and adaptable.
3. Interoperability:
Interoperability is the capability of the various modules and systems to be integrated smoothly. According to standard communication and data formats, different technologies can be easily adopted by organizations. This integration enhances productivity and enhances the output of the two systems.
4. Reinforcement Learning (RL):
RL helps the AI systems learn as they go on improving themselves. Such systems are capable of adapting to the environment they are placed in. This is different from the traditional AI which uses set programming, the RL systems learns with feedback. This adaptation results in enhanced decision-making as well as a more appropriate management of users’ needs.
Therefore, the principles of Agentic AI architecture are combined and provide a powerful concept. This framework promotes innovation, flexibility, and effectiveness. It prepares organizations for the future in the ever-evolving environment.
What Are the Different Types of Agents in an Agentic Architecture?
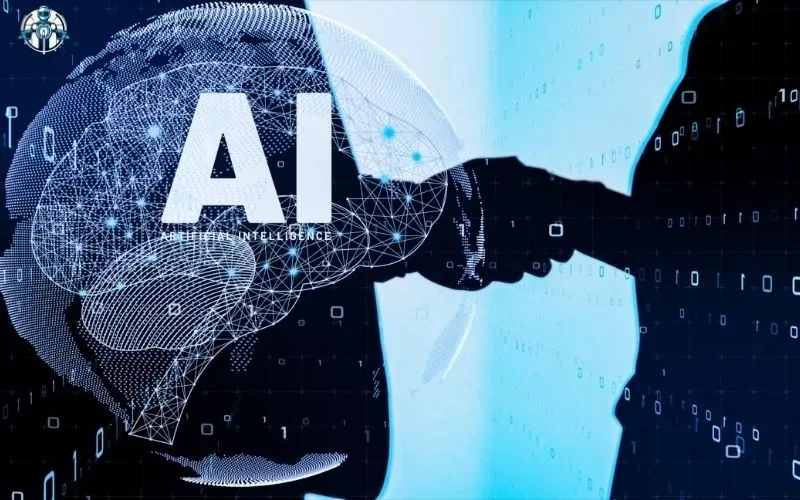
Here are the main types of agents in an agentic architecture:
1. Simple Reflex Agents:
These agents operate on current inputs based on certain rules. For example, a thermostat turns on the heater as soon as the temperature falls to a given level.
2. Model-Based Reflex Agents:
These agents operate with the help of an internal world model, which controls their actions. It can work in dynamic conditions. For instance, a self-driving car uses sensors and maps to move on the roads.
3. Goal-Based Agents:
These agents aim at certain objectives. They consider various steps and select the one that most effectively allows them to achieve their objective. For example, the delivery robot has to look for the best path to take to deliver a package.
4. Utility-Based Agents:
These agents are concerned with the performance of the system with respect to a utility function. They consider the ideal scenarios. For instance, an AI trading system that aims at making revenue by purchasing and selling of stocks.
5. Learning Agents:
These agents are developed in that they adapt as they go through their learning process. They change their ways and work even better. For instance, a recommendation system identifies what a user likes in order to recommend better products.
Curious about how agentic AI systems are built? Dive into the details with our post on What Is the Baseline Architecture of Agentic AI Systems?
What is a Single-Agent System, and How Does it Compare to Multi-Agent Systems?
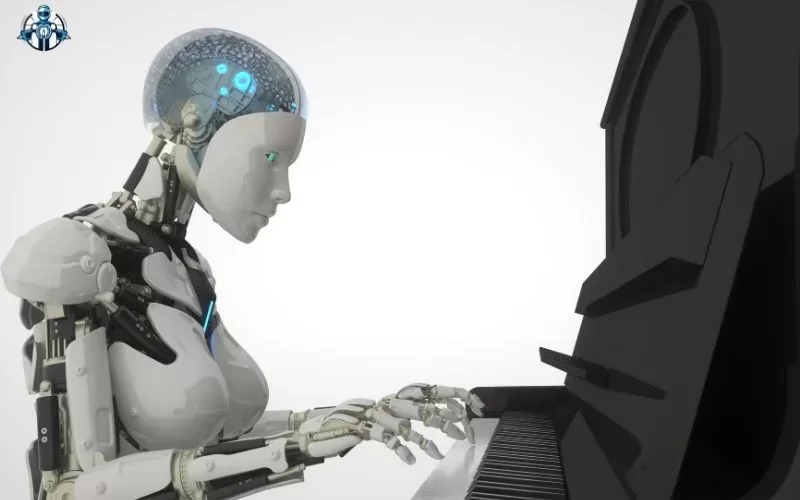
1. Single-Agent System
This type of system entails one artificial intelligence agent who has tools to address certain tasks. This agent can function on his or her own. It applies its equipment and logic to generating and implementing a strategy in a sequence of actions. The agent constructs a plan to accomplish the user’s goal and this can be easy or tough. Then, it employs all the tools they require to accomplish each assignment. Then it is accumulated all the findings of each step to produce the final product of the work.
2. Multi-Agent Systems
On the other hand, multi-agent systems are systems that are made up of multiple agents who interact with one another. These agents communicate with one another and work together in problem-solving. This problem-solving teamwork results in better and compounded solutions.
The Process of Achieving a User’s Goal
In order to get the user to the desired goal, approaches may be different. They are influenced by the tools used, the objectives of the particular assessment, and constraints. Therefore, it is very important to write the prompt very carefully. This careful approach will be used by the agent in its decision-making. It will also guarantee that resources will not be put to waste in an effort to accomplish the goals set.
Why Single-Agent Systems Remain Relevant
Single-agent systems are beneficial in many ways. This is because they have a simple design. So, they are easy to design and organize. The proposed approaches do not require coordination of multiple agents, which simplifies the communication process. This is a big advantage. The application is very simple.
These systems ensure that there is a uniformly good and correct approach to decision-making. When there is one agent in charge, there is no competition of goals and actions among the agents. Therefore, the behaviour of the system can be easily determined. This makes the system quite easily comprehensible and it is easier to locate the problem and rectify it.
Low coordination tasks are well handled by single-agent systems. The goals can be realized when decentralized decision-making is not appropriate.
Limitations of Single-Agent Systems
Single-agent systems have demerits as well. They are usually targeted at certain objectives, which makes flexibility a challenging issue. In a dynamic environment, this can pose a problem because they have a short range of applications. They may have difficulty in places that call for multiple skills.
To make a single agent handle more complicated tasks, it is very often that redesign is needed. It is not enough to add new features. It makes the system less efficient when scaling.
One of the major problems of single-agent systems is that they have limited memory and computational power. Single-agent works on all tasks, which is a disadvantage of the system in terms of resources. This constraint can be counterproductive to the aim of efficiency and effectiveness.
Multi-Agent System
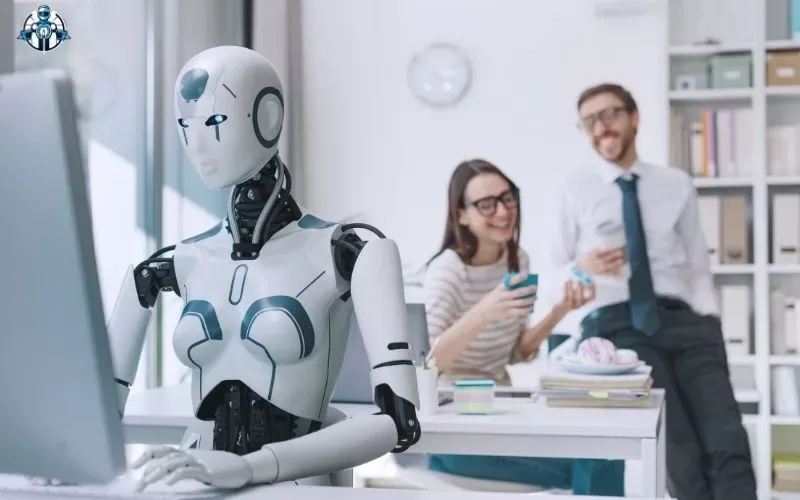
A multi-agent system is a system where a number of agents operate independently of each other. Every agent has the language model to perform a number of tasks that require significant effort. This is unlike the single-agent system, where all the operations are controlled by a single agent. A multi-agent system has each agent with specific abilities that it brings to the system.
This results in efficiency and accurate decision-making. The agents can represent many aspects and can be specialized in different aspects. They also work harmoniously in order to solve problems.
Among the most important benefits of a multi-agent system is the possibility of its extension. If there is an increase in workloads or new tasks to be done, it is very simple to add more agents. This is rather advantageous because the system can easily navigate through new challenges. Moreover, multi-agent systems are tolerant to failure.
In case one agent gets a failure, the other agents will be able to take over the functionality without interruption. So, a multi-agent system enhances the way we address dynamic and composite tasks by delegating responsibilities, coordinating work, and handling failure.
How Have Large Language Models (LLMs) Influenced the Rise of Agentic AI?
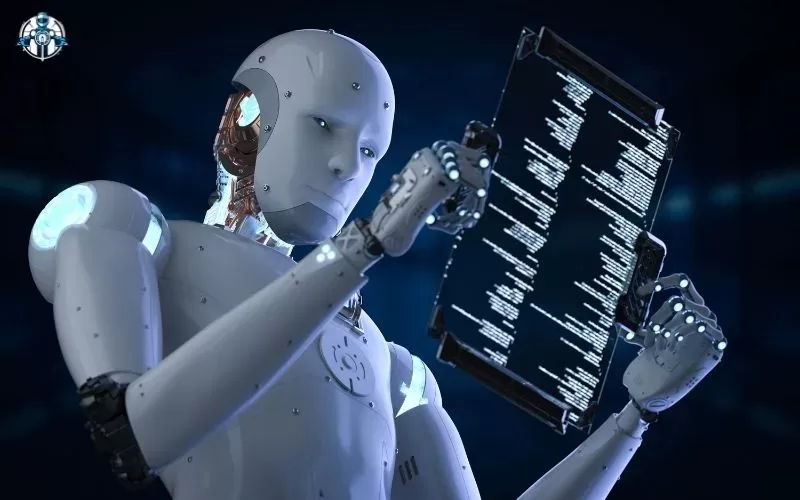
The large language models (LLMs) have been the latest addition to the artificial intelligence field. Many models including GPT-3 and GPT-4 have been trained on large data sets. They can get the meaning of the text, which imitates human language, and generate texts that may seem like written by a person. That is why the interaction with AI becomes more like a conversation. This has led to the generation of many opportunities in the application of AI.
LLMs are essential to what is known as “agentic” AI. This type of AI is self-sufficient, that is it can work on its own. These models allow the AI agents to understand the given instructions, interact coherently, and create new text. AI communication has been improved to make it easy for it to interact with the user, improving teamwork and problem-solving.
In addition, LLMs allow agentic AI to analyze a vast amount of data and find correlations. This enables them to reason, decide, and act in a manner that will support certain goals and objectives. The potential to think and act on their own is what defines agentic AI from other forms of automation methods.
Confused about how agentic AI compares to large language models? Read our blog on What is the Difference Between LLM and Agentic AI? to clear up the confusion
What Machine Learning Advances Have Fueled Agentic AI, and How?
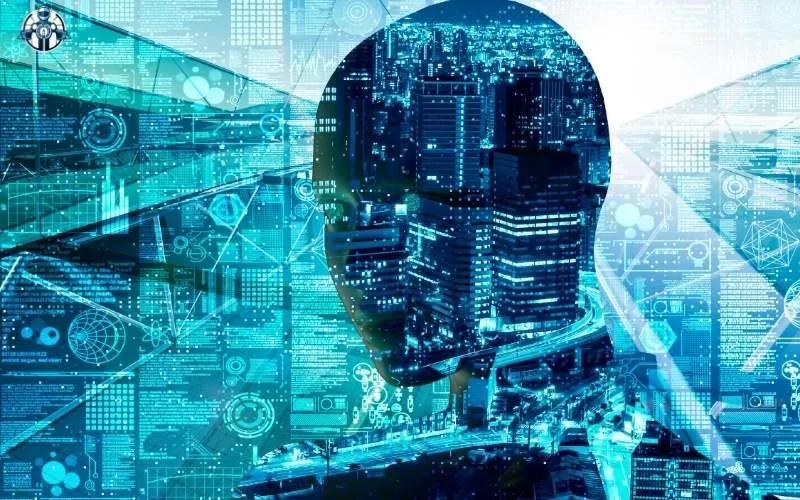
This has been due to the enhanced machine learning algorithms and the increased computing power. These systems now work with big data more effectively than ever before. They are able to acquire further knowledge and should be able to cope with new situations better. This development results into better and more versatile automation of several tasks and more extensive use of AI.
One key example is reinforcement learning. In this approach, AI agents learn by interacting with their environment and getting feedback. This method allows AI to make smart choices. It learns from failures and improves its actions to achieve goals. This is especially useful in complex and shifting situations.
What Technological Innovations Are Driving the Use of Agentic AI in Enterprises?
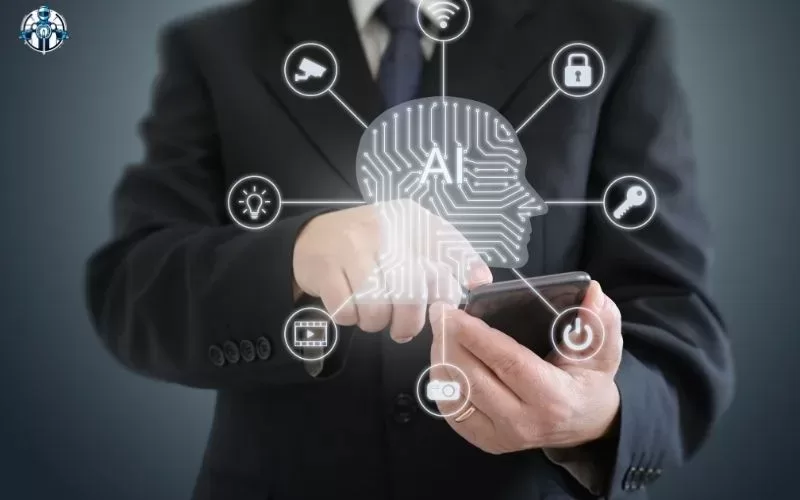
Integrating AI tools with existing systems has changed businesses greatly. AI agents now access a wide range of data across departments like CRM, ERP, supply chain, and HR. This integration breaks down data silos. As a result, AI gains a complete view of the organization, which enhances decision-making and automates complex tasks.
Advancements in cloud computing also play a significant role. Platforms like Microsoft Azure and AWS provide the resources needed for training and using advanced AI models. These platforms also offer strong data processing tools. They enable real-time analysis of large data sets. This greatly improves AI’s decision-making abilities.
Plus, AI-driven automation solutions have boosted the use of agentic AI. These platforms make it simple to create and manage intelligent agents. These agents can perform many complex tasks. Hence, businesses find it easier to implement AI-driven automation.
Discover how agentic AI is reshaping security operations in our blog: How Is Agentic AI Transforming SOC Teams in 2025?
What Are the Advantages of Agentic AI and Agentic Automation?
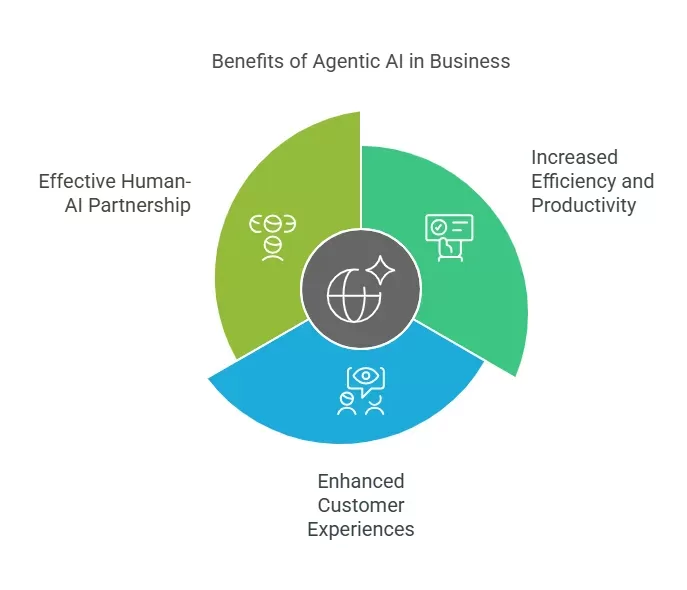
Agentic AI refine the capability of AI systems, that is, they make AI systems capable of performing more complex tasks. This change enhances the chances of automation and enhances the ways through which AI agents engage the human. To the businesses, these are the following advantages that come with these changes.
· Increased efficiency and productivity
An empowered software agent can handle many tasks that involve a lot of decision-making. This frees up people to do what they really should be doing, which is strategic thinking and creative decision-making. They also help improve customer relationships. All these actions spur business growth.
· Enhanced customer experiences
Agentic AI results in altering the manner in which organizations engage their customers by offering tailored and timely responses. With the help of technology, AI agents comprehend the needs of the customers. It can mean that they can identify requirements and provide specific services. They operate without stopping to guarantee stable and practical support at all times.
· Effective Human-AI partnership
In particular, the agentic AI systems are implemented as assistants to human workers rather than substitutes for them. It improves human performance, efficiency and motivation. Such systems are also easily compatible with other workflow systems in use. This collaboration makes AI and workers to have a good relationship where companies can address various challenges, make decisions and increase productivity.
For instance, in the production sector, agentic AI can manage an entire production process. It corrects activities on the fly thus eliminating delays or errors which may occur. This is not only economical but also makes the product better in quality.
Want to understand agentic automation? Read our simple guide: What is Agentic Automation?
Why Agentic AI Is the Future of AI Research
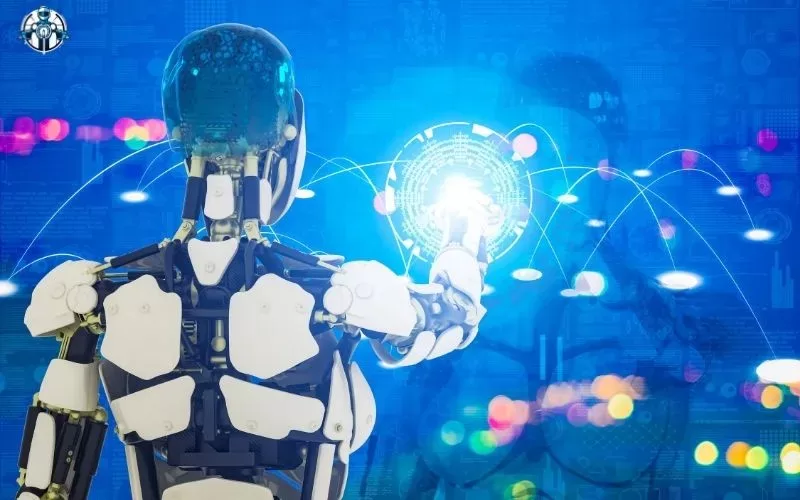
The excitement about agentic AI, particularly Large Language Models (LLMs), is justified for several reasons:
1. Flexibility and Precision:
LLMs allow users to interact using natural language, understanding complex contexts and generating creative content. While traditional programming is reliable and methodical, agentic AI combines LLMs’ adaptability with traditional programming’s precision for intuitive decision-making in dynamic environments.
2. Extended Reach:
LLMs operate on fixed datasets and cannot update knowledge post-training. In contrast, agentic AI actively collects real-time information from the web and APIs, managing tasks like data collection and trend analysis. This ongoing feedback helps refine models and strategies.
3. Autonomous:
Powered by advanced LLMs, autonomous AI can perform tasks independently, reducing the need for human oversight. It can manage marketing campaigns, analyze patient data in healthcare, monitor cybersecurity threats, optimize supply chains, and personalize training in HR.
4. Intuitive:
Agentic systems can simplify business tasks currently done via SaaS products by allowing employees to interact using natural language. For example, project management tools could streamline information retrieval, significantly reducing manual effort in tasks like creating presentation slides.
Overall, agentic AI enhances efficiency and effectiveness across various fields.
What Does the Future Hold for Agentic AI, Agentic Automation, and AI Agents?
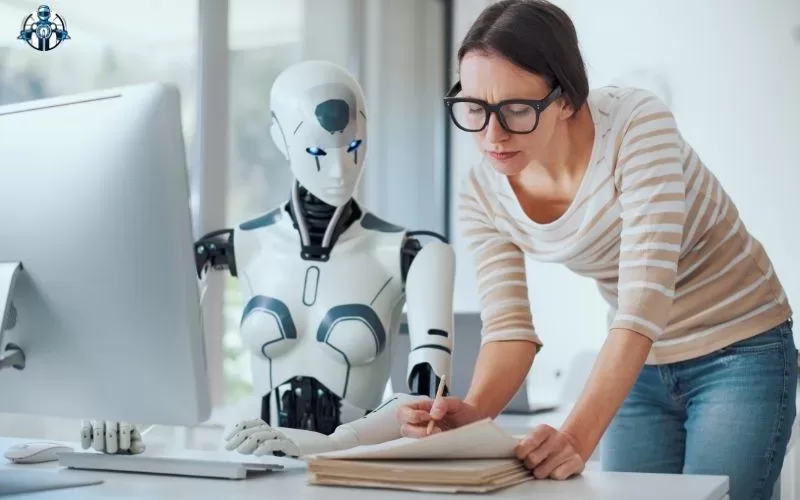
The future of agentic AI, agentic automation, and AI agents will bring big changes to many industries. By 2025, these technologies will shift from performing single tasks to working together in connected systems. Multi-agent systems will drive collaboration to tackle complex issues.
In healthcare, agentic AI will take over important duties. It will schedule appointments, check on patients, and create personalized treatment plans. This change will improve efficiency and reduce mistakes. In logistics, AI agents will predict demand and find better routes. They will also manage inventory in real-time and give useful instructions to workers.
The rise of small language models will help adopt agentic AI more widely.
Experts predict that by 2028, AI agents will autonomously make 15% of daily work decisions. This shift is expected to occur alongside a significant increase in the integration of AI into enterprise software applications, which will rise to 33% from less than 1% today.
So, agentic AI will handle repetitive tasks. This shift will let people focus on creative and strategic work. As a result, productivity and innovation will grow across different sectors.
Interested in building autonomous systems? Find out how agentic AI can help in our post: How Can Agentic AI Help Build Autonomous Systems from Scratch?.
What Are the Most Impactful Uses of Agentic AI Today?
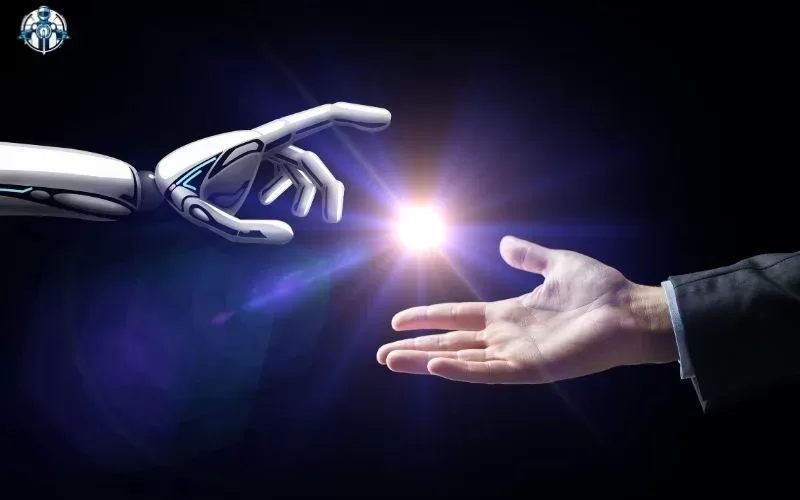
Agentic AI is making a big difference in many areas:
· Insurance Claims Processing:
Agentic AI speeds up the insurance claims process. It reduces paperwork and cuts down on mistakes. It checks claims, collects needed information, and talks directly with customers. This helps resolve claims faster and allows human adjusters to deal with more complicated issues.
· Logistics and Supply Chain Management:
In logistics, time is essential. Agentic AI looks at real-time data to find the best delivery routes. It can predict delays and adjust inventory as needed. As a result, operations become more efficient, costs go down, and customer satisfaction improves.
· Financial Decision-Making:
In finance, agentic AI makes things better by spotting market trends. It helps create personalized financial plans for clients. Financial advisors can focus more on building relationships while identifying risks. This proactive approach helps prevent losses.
Learn how agentic AI is set to change the financial industry in our article: How Will Agentic AI Revolutionize Financial Services?
· Drug Discovery and Development:
In healthcare, agentic AI personalizes treatment plans and speeds up drug discovery. It quickly analyzes a lot of data. By doing this, it finds potential drug targets and predicts how well they might work. This speeds up research and lowers costs.
· Customer Service:
Agentic AI enhances customer service by providing personalized support around the clock. It understands spoken and written questions. It solves complex problems and customizes responses based on past interactions. This approach increases customer loyalty.
· Integration with IoT:
Combining agentic AI with the Internet of Things helps with real-time monitoring in many industries. This union improves efficiency and safety while also cutting costs.
What Are the Risks and Challenges of Adopting Agentic AI?
While agentic AI provides many advantages, it also brings risks:
- Job Loss: Automation of tasks may result in less employment for people.
- Privacy Concerns: AI systems require data to function, which raises privacy issues.
- Bias: If the information applied to an AI is flawed, then the classifier will make prejudiced decisions. For example, an AI system for recruitment may discriminate against some groups of people if the input data is biased.
Thinking about adopting agentic AI? Learn about the risks in our blog: What Should Enterprises Know About Agentic AI Risks?
What Are the Best Practices for Implementing Agentic AI?
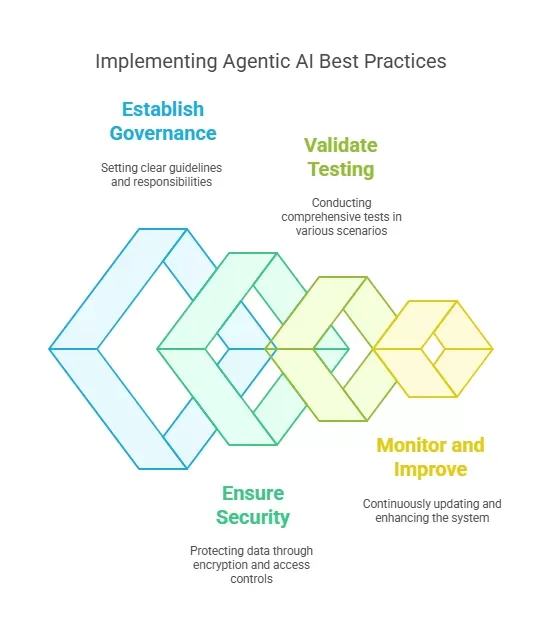
To implement agentic AI successfully, businesses should follow these best practices:
· Strong Governance
Put down guidelines and procedures clearly. Establish duties in the creation of autonomous AI systems and the use of the same. Provide recommendations for the use of AI. Comply with all laws and regulations. Also, you should include checks and evaluations on a regular basis to ensure the standards are met to the maximum.
· Security and Compliance
Only with effective security measures it is possible to protect the important data from being compromised. This includes:
1. Encryption: Data security is done by encoding the data into codes. This helps in avoiding exposure to the system by unauthorized people.
2. Access Controls: Restrict access on any information that is available in the system depending on user’s authorization.
3. Regular Vulnerability Assessments: All the time look for where there are loopholes in the system. All the problems should be solved immediately to ensure that the security is not compromised.
· Testing and Validation
Testing and validation are essential for ensuring agentic AI systems are dependable and safe. It is vital to perform comprehensive tests in various situations. You should examine both expected and unexpected scenarios. This approach helps identify and resolve any issues or unintended outcomes before using the system in real-world settings.
· Continuous Monitoring and Improvement
Agentic AI systems require regular updates to stay effective and secure. You can achieve this by gathering ongoing feedback, measuring performance, and considering user input. These steps help identify areas for improvement. By continuously learning and evolving, these systems can adjust to new circumstances. This ultimately enhances their value and extends their lifespan as important tools.
Planning to invest in AI? Check out our list of Top 5 Agentic AI Stocks to Watch in January 2025 for insight
FAQs
What is Agentic AI?
Agentic AI describes artificial intelligence systems that can operate on their own to reach specific goals.
Who is the leader in Agentic AI?
Forethought leads the way in using AI to enhance customer experiences. They manage over one billion customer interactions each month. This demonstrates that Forethought plays a significant role in changing customer experiences by combining AI automation with human assistance.
What is the Difference Between Generative AI and Agentic AI?
Generative AI makes new content such as text or images. In contrast, agentic AI takes actions to achieve specific goals.
How is Agentic AI Different from RPA?
RPA, or Robotic Process Automation, follows strict rules set by programmers. On the other hand, agentic AI can make choices and adapt to new challenges.
What is the Difference Between LLM and Agentic AI?
Large Language Models, or LLMs, can read and write text. Conversely, agentic AI acts based on what it learns.
Which Type of LLM is the Best?
The best LLM depends on the job. For instance, GPT-4 excels at generating text, while BERT is more suited for understanding language.
What is the Difference Between AGI and Agentic AI?
AGI, or Artificial General Intelligence, aims to match human intelligence in all areas. Meanwhile, agentic AI focuses on making decisions and taking action within specific tasks. AGI is broad, while agentic AI is task-focused.
What are the Concerns of Agentic AI?
Key concerns include potential job losses due to automation, privacy risks from data collection, biases in decision-making, and the chance of AI systems making mistakes or acting unpredictably in complicated situations.
What are the 4 Main Agentic Features of Human Agency?
The four main features of human agency are:
1. Intentionality: Creating plans and strategies to reach goals.
2. Forethought: Setting goals and predicting outcomes to shape actions.
3. Self-reactiveness: Adjusting actions based on personal standards and self-checking.
4. Self-reflectiveness: Considering thoughts, actions, and success to make improvements.
What is Agentic Process Automation?
Agentic process automation uses AI to handle complex tasks that involve decision-making.
What Are the Skills of Agentic AI?
Agentic AI can learn and adjust. It can also make decisions and take actions to meet its goals.
Final Words
As you learned, agentic AI is changing technology by making machines smarter and more independent. It impacts many fields, from healthcare to transportation. While challenges exist, the advantages of agentic AI are significant. As we keep developing this technology, we can expect even more exciting improvements ahead. So, by understanding agentic AI and its functions, we can prepare for a future where machines serve as partners in solving problems and enhancing our lives.